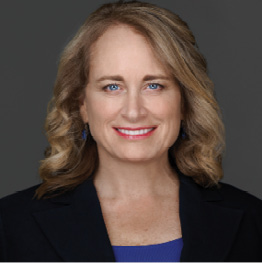
ChatGPT recently “passed” the US Medical Licensing Exam with a 60% grade. This accomplishment marks the first time AI has achieved a passing score and introduces the idea that AI may be used as a medical education or diagnostic tool.1 AI, however, is not yet prepared to assume patient care responsibilities. A California ER doctor experimented with ChatGPT as a diagnostic tool and found that his diagnoses for approximately 40 patients aligned with one of the six differential diagnoses generated by ChatGPT about half of the time.2 Of concern, ChatGPT missed some potentially life-threatening diagnoses. Machine learning will nevertheless likely prove to be a valuable diagnostic aid for physicians across various settings and specialties.
AI, machine learning, and big data are some of the forces poised to shape the course of advancement in many different professions, including ophthalmology. Free access to sites such as ChatGPT (OpenAI), Dall-E (OpenAI; Figure), and Jenni AI (Sympli AI) demonstrates in a mind-boggling way the power these tools hold. It also shows the present limitations inherent in AI when human input is minimized. The output quality depends more on asking the right questions than knowing all the answers. Having experimented with these websites, I am impressed by the speed at which data are accessed. The quality of the data and the ranking of its importance and relevance, however, are limited. Perhaps I am simply not asking the right questions or guiding with the right parameters.
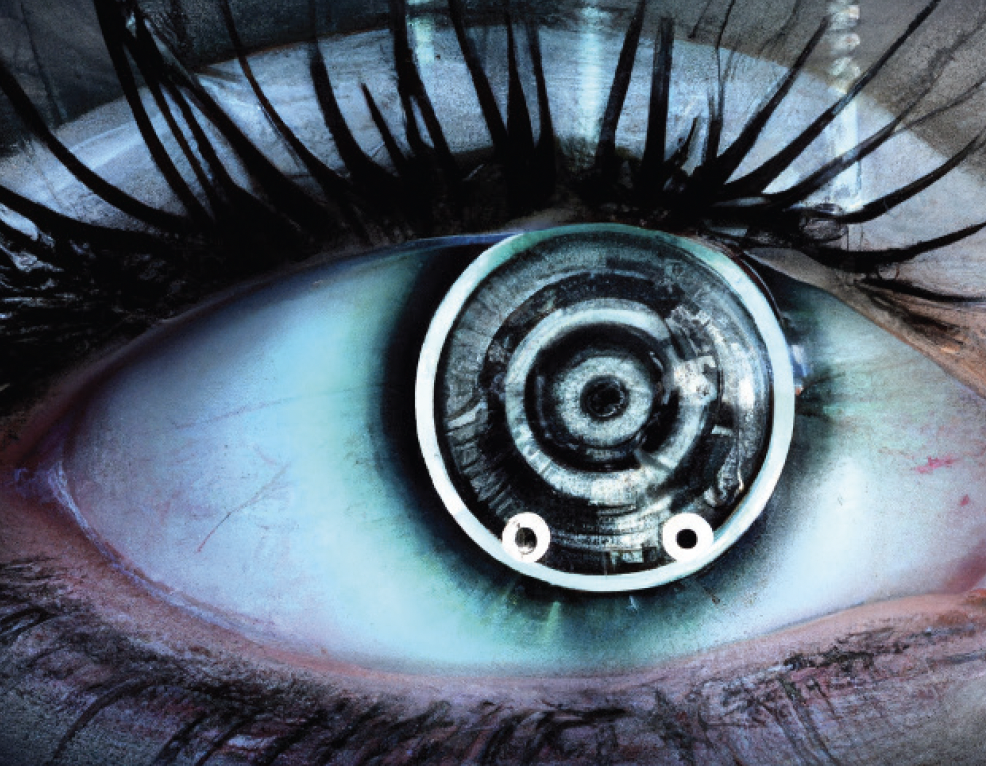
Figure. Image generated with Dall-E from the prompt “the challenges of artificial intelligence and big data in ophthalmology” illustrating the intricacies involved in these cutting-edge technologies.
Machine learning and big data analysis promise refined, accurate, and applicable trends and truths, even at the fringes of the bell curve. Continual or frequent refinements in nomograms and data processing based on big data feedback loops may bring us closer to optimal diagnoses and treatment plans for various conditions and individuals. For example, eyes with complex corneas such as those with a history of radial keratotomy or LASIK performed in the 1990s and early 2000s make predicting refractive outcomes with current IOL calculation methods challenging. The more data collected from challenging cases and the tighter the feedback loop for refining nomograms, the more accurate the outcomes. The quality of data used to close the loop, however, and its application to individuals will continue to depend on human evaluation.
Hard and soft data points influence the best approach to complex corneal cases. Higher-order aberration measurements, epithelial mapping, and anterior segment OCT imaging to determine corneal scar depth are important. Truly individualized treatment planning, however, requires a more holistic patient assessment, including their needs and desires, their ability to comply with postoperative care recommendations, the health of the fellow eye, and their social situation. The art of medicine encompasses all these details. Only when it is combined with surgeon skill, experience, and comfort level can a treatment plan truly be tailored to an individual patient. AI can help identify patterns in a large volume of data, but the final decision relies on the unique relationship between the patient and the surgeon. Ultimately, the quality of the questions we ask of machine learning tools and our patients will help us provide the best, most personalized outcomes.
Cathleen M. McCabe, MD | Chief Medical Editor
1. Kung TH, Cheatham M, Medenilla A, et al. Performance of ChatGPT on USMLE: potential for AI-assisted medical education using large language models. PLoS Digit Health. 2023;2(2):e0000198.
2. Tamayo-Sarver J. I’m an ER doctor: here’s what I found when I asked ChatGPT to diagnose my patients. Fast Company. March 13, 2023. Accessed March 15, 2023. https://www.fastcompany.com/90863983/chatgpt-medical-diagnosis-emergency-room