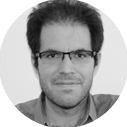
Artificial intelligence has the potential to facilitate the diagnosis and management of glaucoma. In low- to middle-income countries where access to eye care is limited, the technology could be a game-changer for the early detection of the disease. Current AI tools could be used to enhance the screening, diagnosis, and monitoring of glaucoma and eliminate the subjective errors associated with current methods of diagnostic testing.1
Globally, nine out of 10 people with vision loss live in low- and middle-income countries where quality eye care is often difficult (and sometimes impossible) to access. The opportunities for technology to make a difference in these areas are profound for patients and health care providers alike. This article reviews the developing role of AI in glaucoma and describes how the technology could alter care in the future.
A COMPLEX DIAGNOSIS
Glaucoma is complex by nature: It is a group of eye conditions associated with vastly different and potentially subtle symptoms. The complexity is reflected in diagnosis, which typically relies on a combination of tests, such as optic nerve head assessment and photography, OCT imaging, visual field assessment, and IOP measurement. The interpretation of the various test results can be challenging and requires extensive knowledge and experience. Further, the range of symptoms and testing modalities makes it difficult to standardize the diagnosis of glaucoma through universal grading schemes or severity scales.
These challenges are compounded in low-resource environments. An acute shortage of eye care specialists means that some patients do not get an opportunity to be diagnosed, let alone treated. The equipment needed to perform the aforementioned tests may be unavailable, resulting in a less accurate diagnosis. These are significant hurdles to tackling glaucoma in low- and middle-income countries, and they preclude the establishment of successful screening programs like those established for other conditions such as diabetic retinopathy (DR).
A PLAUSIBLE SOLUTION
In the past few years, advances in AI have enabled the development of algorithms that match or arguably exceed human performance in interpreting medical data. Ophthalmology was at the forefront of this AI revolution, and DR screening emerged as an early-use case. A study conducted by Orbis showed that DR screenings supported by AI enhanced patient uptake of referral services.2 AI could have similar results when applied to screening for other eye conditions.
The standardized nature of DR screening and the availability of large datasets make it a perfect fit for AI algorithms, which are exceedingly good at detecting predetermined patterns in images when trained with enough data. Significantly less data is available for the diagnosis of glaucoma, and the lack of standardization further complicates the development of robust AI algorithms in this domain. AI applications in glaucoma tend to focus on a single modality, such as optic disc photography; although a useful tool for screening, this modality alone is unlikely to suffice for ascertaining a diagnosis. That said, AI algorithms have been shown to find useful signals that were previously unknown to the most knowledgeable experts. It is possible that an algorithm could perform at an optimal level with a single modality, but this has yet to be demonstrated in real-world applications for glaucoma.
Developments in AI could have a significant impact on the ability to minimize subjectivity in the diagnosis of glaucoma. For example, multimodal approaches can combine different types of data (eg, photographs, OCT scans, visual field tests, and patient history) similarly to how a glaucoma specialist would integrate information from an examination and testing. Because labeled data (ie, data that have been reviewed and annotated by experts) for glaucoma are scarce, there is also a shift toward semisupervised and unsupervised techniques that can make the most of unlabeled data, which are typically available in much larger quantities but ultimately excluded by more conventional supervised approaches.
For now, it is likely that AI solutions will remain assistive rather than autonomous. As such, they are likely to be deployed alongside clinicians and to play a supporting role in clinical decision-making (see Orbis International Employs AI to Enhance Eye Care). AI-enabled systems can read the data acquired during a patient encounter and provide recommendations and alerts if glaucoma-related markers are detected. I expect the primary result of this AI application to be more objective and uniform clinical outcomes and a reduced risk of interpretation error.
Orbis International Employs AI to Enhance Eye Care
The development of AI algorithms and software is not simple, but the real challenge is understanding how this technology can be deployed safely and ethically to reach the areas where it is needed most. Many technological advances never achieve this outcome.
In an effort to achieve more widespread use, Orbis International provides eye care professionals in low- and middle-income countries with free access to its AI tool, Cybersight AI, available through the organization’s telemedicine and virtual training Cybersight platform. Cybersight AI can quickly detect abnormalities associated with common eye diseases such as glaucoma, diabetic retinopathy, and macular disease by analyzing images taken during routine examinations.
The Cybersight AI tool helps eye care professionals to detect disease and learn how to better identify disease in the future, a concept Orbis refers to as machine mentoring. Eye care professionals who use Cybersight AI can also choose to discuss diagnostic results with an Orbis volunteer faculty member to determine the best course of care.
Orbis’ approach to AI is human-centric. Cybersight AI was not developed to replace eye care professionals but rather to provide them with a tool that enhances their decision-making and supports their efforts to provide the best patient care possible.
AI FOR GLOBAL GLAUCOMA CARE
In low-resource environments, AI solutions may enable nonexpert allied health care professionals to confidently take part in the glaucoma diagnosis and management workflow, helping to break down some of the barriers to eye care access. However, AI-enabled glaucoma diagnostics can be implemented in these regions only if they can accommodate both the shortage of ophthalmologists and the limited availability of medical devices for testing. For example, a solution could be a system capable of reliably screening for glaucoma based on optic disc photographs acquired using a low-cost retinal camera. In many regions, implementation of these solutions may be complicated by issues with computational hardware or internet connectivity, but certain off-the-shelf solutions can be adapted to overcome these obstacles.
AI-based screening tools must be deployed as part of a screening program that also includes provisions for the monitoring and treatment of patients with glaucoma. Identifying patients at risk of glaucomatous progression is important but ultimately fruitless if they cannot get the treatment they need. Effective screening programs prioritize patient outreach and education, and they provide the infrastructure and personnel necessary for these initiatives.
CONCLUSION
In high-income countries, AI will likely make glaucoma screening more robust and widely available outside of specialist care, resulting in incremental improvements in the diagnosis and treatment of the disease. In lower-income countries, AI technology has the potential to establish a pathway for glaucoma diagnosis and monitoring that gives local patients the opportunity to preserve sight through early detection and treatment.
1. Ittoop SM, Jaccard N, Lanouette G, Kahook MY. The role of artificial intelligence in the diagnosis and management of glaucoma. J Glaucoma. 2022;31(3):137-146.
2. Mathenge W, Whitestone N, Nkurikiye J, et al. Impact of artificial intelligence assessment of diabetic retinopathy on referral service uptake in a low resource setting: the RAIDERS randomized trial. Ophthalmol Sci. Published online April 30, 2022. doi:10.1016/j.xops.2022.100168